Overview

ABSTRACT
The concept of the chain was introduced in 1902 by Andrei Markov in order to formalize epistemologic and encryption issues. Later, around the years 1940-1950, a much better and adapted formalism appeared, offering effective operating modes inspired by the general theory of stochastic processes and the potential theory. This presentation is basic and only requires a basic knowledge in probabilities. Examples illustrate the theory leading to generic algorithmic approaches: algorithms for invariant measures, dynamic programming and hidden Markov chains.
Read this article from a comprehensive knowledge base, updated and supplemented with articles reviewed by scientific committees.
Read the articleAUTHOR
-
Jean LACROIX: Professor at Paris VI University
INTRODUCTION
The notion of chain was introduced in 1902 by Andrei Markov to formalize problems of epistemology and encryption.
The state space was then finite, and for a long time many users were content with matrix manipulations, which quickly reached their limits, even with today's computing resources. It wasn't until the 1940s-1950s that a much better adapted formalism appeared, proposing effective operating modes inspired by the general theory of stochastic processes and potential theory. The presentation here is deliberately elementary, requiring only a basic knowledge of probability. Indeed, we restrict ourselves here to a countable state space and make no use of more elaborate concepts such as filtrations or martingale theory. The notion of Markov dependence is very intuitive; on the other hand, computational techniques require more dexterity and training. For this reason, a large number of proofs and examples are provided, to enable the reader to practice manipulating new tools. A few proofs are also written to compensate for the heaviness of certain presentations, mainly those described in paragraph . As far as applications are concerned, which are extremely numerous, we have chosen a few examples which lead to generic algorithmic procedures, relatively recent and in widespread use: algorithms for finding invariant measures (Propp-Wilson, Metropolis), dynamic programming (Bellman) and hidden Markov chains (E.M.).
Exclusive to subscribers. 97% yet to be discovered!
You do not have access to this resource.
Click here to request your free trial access!
Already subscribed? Log in!
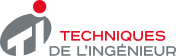
The Ultimate Scientific and Technical Reference
This article is included in
Mathematics
This offer includes:
Knowledge Base
Updated and enriched with articles validated by our scientific committees
Services
A set of exclusive tools to complement the resources
Practical Path
Operational and didactic, to guarantee the acquisition of transversal skills
Doc & Quiz
Interactive articles with quizzes, for constructive reading
Markov chains
Bibliography
References
Exclusive to subscribers. 97% yet to be discovered!
You do not have access to this resource.
Click here to request your free trial access!
Already subscribed? Log in!
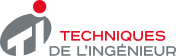
The Ultimate Scientific and Technical Reference