Overview

ABSTRACT
This article is a short introduction to some key concepts in supervised machine learning. It also presents two applications to air traffic management. The first one consists in learning an air traffic controller workload model from recorded aircraft flightpaths and past control sector openings. The second application concerns learning models predicting the altitude of climbing aircraft.
Read this article from a comprehensive knowledge base, updated and supplemented with articles reviewed by scientific committees.
Read the articleAUTHOR
-
David GIANAZZA: Teacher-researcher, qualified to direct research - École Nationale de l'Aviation Civile, Toulouse, France
INTRODUCTION
Field: artificial learning and air traffic management
Degree of technology diffusion: growth
Contact: [email protected]
Artificial learning is a rapidly expanding field of science. With the emergence of massive data (Big Data), it has become an indispensable tool for extracting useful information or predictive models from these data, in many fields of application.
The field of learning can sometimes appear to the neophyte as a veritable jungle, obscure and inaccessible. This article in no way claims to explore it exhaustively, or even superficially. The aim is to provide the reader with an entry point and a few tools (a machete, to continue our analogy), so that he or she can then explore the field on their own, through specialized reading. Reference works include books by T. Hastie et al. , C. Bishop , and in French, the work by A. Cornuejols and L. Miclet .
This introduction to artificial learning is illustrated by two applications to air traffic management problems.
In the first, the workload prediction model for air traffic controllers ("aiguilleurs du ciel") presented is a neural network, learned from aircraft trajectory recordings and control sector opening archives. Once learned, this model is combined with a tree search method to predict optimal air traffic control sector opening configurations.
In the second application, machine learning can be used either to directly predict the altitude of a climbing aircraft, or to estimate certain parameters of the aircraft's physical model, not available in ground-based predictors, in order to improve this altitude prediction. The elements presented in this article are further detailed in
Exclusive to subscribers. 97% yet to be discovered!
You do not have access to this resource.
Click here to request your free trial access!
Already subscribed? Log in!
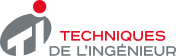
The Ultimate Scientific and Technical Reference
KEYWORDS
Probability | forecast model | neuron network
CAN BE ALSO FOUND IN:
Home Transports Aerospace systems Machine learning and applications to air traffic management
Home Industrial engineering Industry of the future Machine learning and applications to air traffic management
Home IT Software technologies and System architectures Machine learning and applications to air traffic management
Home Innovations Technological innovations Machine learning and applications to air traffic management
This article is included in
Smart cities
This offer includes:
Knowledge Base
Updated and enriched with articles validated by our scientific committees
Services
A set of exclusive tools to complement the resources
Practical Path
Operational and didactic, to guarantee the acquisition of transversal skills
Doc & Quiz
Interactive articles with quizzes, for constructive reading
Artificial learning and its application to air traffic management
Bibliography
Events
USA/Europe ATM R&D seminar
http://www.atmseminarus.org/
International Conference on Research in Air Transportation
http://icrat.org/icrat/
Websites
IEEE Transactions on Intelligent Transportation Systems
Transportation Research
https://www.journals.elsevier.com
MOOC Statistical Learning (Stanford on-line)
Exclusive to subscribers. 97% yet to be discovered!
You do not have access to this resource.
Click here to request your free trial access!
Already subscribed? Log in!
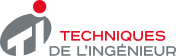
The Ultimate Scientific and Technical Reference