
6. Learning in multi-agent universes
It's not always possible to define completely and precisely, at the design stage, the environment in which an agent will evolve, nor the coordination rules of an ADM. It is also difficult to optimize agent behavior a priori, given the open, evolving and unpredictable nature of the environment and the complexity of the situations they may encounter. Machine learning enables an agent to gradually discover and adapt to its environment, to become more efficient or to learn how to coordinate with its associates to carry out a complex task. The example of a team of soccer robots is a case where learning enables a team to establish strategies, each teammate to learn to play a role (goalkeeper, defender, attacker, midfielder) in the service of the common goal (winning a match) and to adapt collective behavior to the opposing team, whose behavior and organization are not known a priori.
...Exclusive to subscribers. 97% yet to be discovered!
You do not have access to this resource.
Click here to request your free trial access!
Already subscribed? Log in!
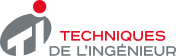
The Ultimate Scientific and Technical Reference
This article is included in
Control and systems engineering
This offer includes:
Knowledge Base
Updated and enriched with articles validated by our scientific committees
Services
A set of exclusive tools to complement the resources
Practical Path
Operational and didactic, to guarantee the acquisition of transversal skills
Doc & Quiz
Interactive articles with quizzes, for constructive reading
Learning in multi-agent universes
Bibliography
Exclusive to subscribers. 97% yet to be discovered!
You do not have access to this resource.
Click here to request your free trial access!
Already subscribed? Log in!
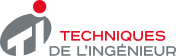
The Ultimate Scientific and Technical Reference