Overview

ABSTRACT
Numerical simulation has become a technique widely used by engineers to design, optimize and qualify numerous products and systems. Resulting from academic and industrial developments, this technique constantly improves its performances. More and more frequently, it benefits from research and innovation in machine learning. This article provides a brief overview of the coupling of these two numerical techniques and their possible uses and applications.
Read this article from a comprehensive knowledge base, updated and supplemented with articles reviewed by scientific committees.
Read the articleAUTHOR
-
Jean-François SIGRIST: Research engineer, science journalist - eye-π – 4, place Foire-le-Roi – 37000 Tours – France
INTRODUCTION
Numerical simulation has become widely used in industry over the past two decades, and is now used in a wide range of engineering applications (mechanical, thermal, acoustic, hydrodynamic, etc.). It benefits from constant innovations stemming from academic research in a variety of fields (physical modeling, applied mathematics, computer science and algorithms, etc.): simulations can account for increasingly complex physical phenomena (such as multiphysical couplings, non-linear behavior, etc.) with growing precision and efficiency. Calculations help to optimize the design of many products and improve their reliability and durability. However, numerical simulations have a number of limitations that restrict their use in certain cases, particularly in terms of robustness, computational resource requirements (computing, storage, etc.) and energy consumption.
Alongside numerical simulation, machine learning techniques are developing, with highly interesting predictive capabilities: based on the growing availability of data (from test results, measurements, sensors, calculations, etc.), machine learning algorithms can be used to build numerical models to complement the models used for physics simulation.
This article, aimed primarily at young engineers and researchers in numerical simulation, offers a brief state-of-the-art on the coupling between numerical simulation and machine learning techniques, which is becoming one of the most interesting avenues for overcoming certain current computational limitations and taking numerical simulation to the next level.
Readers will find these references in the "Further reading" section of this article. An additional bibliography and links to websites provide useful resources for furthering knowledge on the subject.
Exclusive to subscribers. 97% yet to be discovered!
You do not have access to this resource.
Click here to request your free trial access!
Already subscribed? Log in!
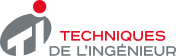
The Ultimate Scientific and Technical Reference
KEYWORDS
artificial intelligence | numerical simulation | computational science | machine learning | mathematical modelling
This article is included in
Management and innovation engineering
This offer includes:
Knowledge Base
Updated and enriched with articles validated by our scientific committees
Services
A set of exclusive tools to complement the resources
Practical Path
Operational and didactic, to guarantee the acquisition of transversal skills
Doc & Quiz
Interactive articles with quizzes, for constructive reading
Numerical simulation and machine learning
Bibliography
Books and articles
Software tools
ABAQUS
ANSYS
CASTEM
Code_Aster...
Directory
Laboratories, engineering schools, universities (non-exhaustive list)
Supelec power plant
https://www.centralesupelec.fr
École Centrale de Nantes
ENSEIRB-MATMECA
Exclusive to subscribers. 97% yet to be discovered!
You do not have access to this resource.
Click here to request your free trial access!
Already subscribed? Log in!
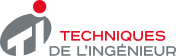
The Ultimate Scientific and Technical Reference