Overview

ABSTRACT
This article deals with supervised statistical machine-learning as a tool for engineers. First, the main theoretical and methodological principles are briefly presented and explained. Then, the article presents the most common models and algorithms used for supervised learning: the main “classical” techniques (Multi-Layer Perceptron, Support Vector Machines, Decision Trees and Random Forests, Boosting) are explained, as well as deep-learning with Convolutional Neural Networks.
Read this article from a comprehensive knowledge base, updated and supplemented with articles reviewed by scientific committees.
Read the articleAUTHOR
-
Fabien MOUTARDE: Professor - Center de Robotique, Mines ParisTech, PSL Université, Paris, France.
INTRODUCTION
The aim of this article is to present the main principles of supervised statistical artificial learning, as well as an overview of the main algorithms and techniques in this field.
It is these statistical learning techniques that have made it possible to automate increasingly complex tasks, and thus enabled the development and deployment in everyday life of applications such as :
automated handwriting recognition, e.g. for bank cheques and addresses on envelopes;
voice recognition, particularly for automatic telephone systems;
recommendation systems" capable of proposing personalized selections (i.e. adapted to individual tastes and/or interests) of music, videos or information.
Artificial learning is also at the heart of future, even more sophisticated systems such as :
intelligent" robotics (as opposed to the robot-automats of the 1970s, which performed identical repetitive actions);
automated driving of driverless cars;
conversational agents" and automated translation, etc.
In addition, the increasing "digitization" of the world, and the resulting avalanche of data, has meant that over the last twenty years or so, statistical learning has become increasingly popular, covering an ever wider field. Indeed, as information from virtually all sectors (human activities such as commerce, the economy, culture, leisure, etc., but also health and the environment) is increasingly encoded, recorded and transmitted in computerized form, it is becoming possible to access, at virtually no cost, a vast quantity of data to which statistical analysis can be applied, and hence empirical modeling by artificial learning. Last but not least, learning algorithms have made significant progress over the same period, and even more so over the last five years with the development of deep learning, enabling a wide range of engineering problems to be addressed with "industrializable" performance.
Note: at the end of the article, readers will find a glossary of important terms and expressions, as well as a table of acronyms, notations and symbols used throughout the article.
Exclusive to subscribers. 97% yet to be discovered!
You do not have access to this resource.
Click here to request your free trial access!
Already subscribed? Log in!
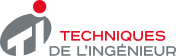
The Ultimate Scientific and Technical Reference
KEYWORDS
classification | regression | empirical model | statistics
This article is included in
Software technologies and System architectures
This offer includes:
Knowledge Base
Updated and enriched with articles validated by our scientific committees
Services
A set of exclusive tools to complement the resources
Practical Path
Operational and didactic, to guarantee the acquisition of transversal skills
Doc & Quiz
Interactive articles with quizzes, for constructive reading
Supervised Statistical Learning
Bibliography
Software tools
For "classic" statistical learning algorithms, the richest and most widely used software tool (containing implementations of most models and algorithms) is :
Sci-Kit Learn (Python library), http://scikit-learn.org
For deep learning of convolutional networks, the main libraries used (all of which integrate...
Exclusive to subscribers. 97% yet to be discovered!
You do not have access to this resource.
Click here to request your free trial access!
Already subscribed? Log in!
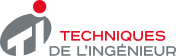
The Ultimate Scientific and Technical Reference