Overview

ABSTRACT
This article deals with physics-informed machine learning, which is a technique leveraging prior physical knowledge and data to describe physical processes. These hybrid models enable to accelerate numerical simulations, to be more data efficient when learning, to provide more interpretable predictions compliant with physical laws that generalize better. This article also presents an industrial application at EDF on short-term solar energy forecasting based on ground-based cameras.
Read this article from a comprehensive knowledge base, updated and supplemented with articles reviewed by scientific committees.
Read the articleAUTHOR
-
Vincent LE GUEN: Researcher - EDF R&D, Chatou - SINCLAIR AI Lab, Palaiseau
INTRODUCTION
The development of computer science and artificial intelligence (AI) has profoundly changed scientific research over the course of the 20th century. The traditional paradigm of theoretical equations and experimental validation has been supported by the use of numerical simulation, which has become essential for analyzing complex systems in physics, engineering, biology and other fields.
With the automation of experiments and the exponential increase in the number of sensors, a deluge of observational data is now available. To extract relevant information from this data and fuel scientific discovery, statistical learning (machine learning), particularly deep learning, is particularly attractive. Over the past decade, deep learning has enabled spectacular progress in the fields of computer vision, natural language processing and gaming, thanks to its ability to extract complex non-linear relationships from massive data and build predictive models. In the industrial field, these AI methods open the way to the development of digital twins, enabling complex systems to be simulated much more rapidly than with traditional solving techniques, and results to be extrapolated to new configurations.
However, deep learning methods require very large, high-quality labeled databases for training, which is not always possible for some very expensive scientific experiments. In addition, purely data-driven methods are often regarded as poorly explainable black boxes, suffer from generalization problems outside their training domain and can produce physically inconsistent predictions.
Introducing physical knowledge into learning methods is a very promising way of solving these problems. Physics-inspired machine learning can be defined as a paradigm aimed at building models that exploit both observational data and a priori physical knowledge to solve tasks that are based on an underlying physical process. These ideas of hybridization are quite old, but have seen a strong revival with the successes of modern deep learning.
This article reviews current knowledge on methods for hybridizing statistical learning and a priori knowledge, focusing on the study of physical phenomena. The main hybridization strategies are presented, and the advantages and fields of application of these methods are discussed. The article also presents an industrial application at EDF for forecasting photovoltaic production from ground cameras, for which a hybrid deep learning model has been developed. Finally, the article looks at the main scientific and industrial challenges ahead for these methods.
Exclusive to subscribers. 97% yet to be discovered!
You do not have access to this resource.
Click here to request your free trial access!
Already subscribed? Log in!
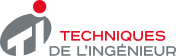
The Ultimate Scientific and Technical Reference
KEYWORDS
physics | deep learning | machine learning | hybrid models
This article is included in
Software technologies and System architectures
This offer includes:
Knowledge Base
Updated and enriched with articles validated by our scientific committees
Services
A set of exclusive tools to complement the resources
Practical Path
Operational and didactic, to guarantee the acquisition of transversal skills
Doc & Quiz
Interactive articles with quizzes, for constructive reading
Physics-inspired statistical learning
Bibliography
Exclusive to subscribers. 97% yet to be discovered!
You do not have access to this resource.
Click here to request your free trial access!
Already subscribed? Log in!
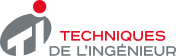
The Ultimate Scientific and Technical Reference