Overview

ABSTRACT
Essential for a good adoption, as well as for a wise and unbiased use, explicability is a real technology lock to
the evolution of Artificial Intelligence (AI), in particular concerning Machine and Deep Learning.
Without an effective explicability of the proposed algorithms, these techniques will remain a black box for users. Increasingly, engineers and designers of AI tools will have to demonstrate their responsibility by providing algorithms that guarantee the explicability of the proposed models. This article presents the motivations of an explainable AI, the main characteristics of the conceptual landscape of explainability in AI, the major families of explainability methods - with a focus on some of the most common methods, to finally present some of the opportunities, challenges and perspectives of this exciting field of human-machine interaction.
Read this article from a comprehensive knowledge base, updated and supplemented with articles reviewed by scientific committees.
Read the articleAUTHORS
-
Daniel RACOCEANU: University Professor, HDR, PhD, M.Sc, Dipl.Ing. - Sorbonne University, Brain Institute – Paris Brain Institute – ICM, CNRS, Inria, Inserm, AP-HP, Hôpital de la Pitié Salpêtrière, F-75013, Paris, France
-
Mehdi OUNISSI: Researcher, M. Sc. - Sorbonne University, Sorbonne Center for Artificial Intelligence (SCAI), Institut du Cerveau – Paris Brain Institute – ICM, CNRS, Inria, Inserm, AP-HP, Hôpital de la Pitié Salpêtrière, F-75013, Paris, France
-
Yannick L. KERGOSIEN: Honorary University Professor, HDR, MD - University of Cergy-Pontoise, Cergy, France
INTRODUCTION
Modern Artificial Intelligence (AI) has experienced unprecedented growth over the past decade. These revolutionary technologies are giving new impetus to many application areas. However, the adoption of these techniques is very often limited by the lack of traceability and feedback for experts. Experts are frustrated by this lack of feedback, even though the very implementation of the tool requires them to make a considerable effort to formalize and make available a colossal amount of expertise. Some authors therefore speak of a "black-box evolution", which is undesirable for the traceable, interpretable, explicable and, ultimately, responsible use of these tools.
The need for explanations of how an intelligent system operates is all the greater when the system's performance exceeds – at least in one specialized domain – human capabilities, and this issue has been addressed since the days of expert systems. Recent deep learning systems (Deep Learning – DL) can achieve astonishing levels of performance, and their large number of parameters makes it all the more difficult to understand the solutions they arrive at, even if these parameters are all accessible. However, the topicality of the subject of explainability for intelligent systems stems less from real breakthroughs – still awaited – in the resolution of this problem than from the legal novelty – which is being imposed in particular on AI players – that constitutes the inclusion in the General Data Protection Regulation (RGPD, European regulation) of explanation obligations for the automatic processing of personal data. We therefore adopt a traditional technological approach to a highly mobile field and propose, alongside examples, a conceptual framework guiding the practitioner's approach to finding solutions.
Exclusive to subscribers. 97% yet to be discovered!
You do not have access to this resource.
Click here to request your free trial access!
Already subscribed? Log in!
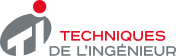
The Ultimate Scientific and Technical Reference
KEYWORDS
machine learning | deep learning | explainable artificial interlligence |
This article is included in
Software technologies and System architectures
This offer includes:
Knowledge Base
Updated and enriched with articles validated by our scientific committees
Services
A set of exclusive tools to complement the resources
Practical Path
Operational and didactic, to guarantee the acquisition of transversal skills
Doc & Quiz
Interactive articles with quizzes, for constructive reading
Explicability in Artificial Intelligence; towards Responsible AI
Bibliography
- (1) - WANG (J.) et al - Learning Credible Models. - In : Proceedings of the 24th ACM SIGKDD International Conference on Knowledge Discovery & Data Mining (juill. 2018). arXiv : 1711.03190, p. 2417-2426. doi : 10.1145/3219819. 3220070. URL : http://arxiv.org/...
In the Laws section
Proposal for a REGULATION OF THE EUROPEAN PARLIAMENT AND OF THE COUNCIL ESTABLISHING HARMONIZED RULES FOR ARTIFICIAL INTELLIGENCE (ARTIFICIAL INTELLIGENCE LEGISLATION) AND AMENDING CERTAIN UNION LEGISLATIVE ACTS. Brussels, 21.4.2021.
Websites
Royal Society – Projects
https://royalsociety.org/topics-policy/projects
IBM-Explainable AI
https://www.ibm.com/fr-fr/watson/explainable-ai
Kaggle
Exclusive to subscribers. 97% yet to be discovered!
You do not have access to this resource.
Click here to request your free trial access!
Already subscribed? Log in!
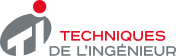
The Ultimate Scientific and Technical Reference