Overview

ABSTRACT
A considerable body of research has been devoted to the design of classifiers whose operating environments are supposed to be static. However, everything that exists changes over time. Thus, classifiers need to adjust their parameters and structure in order to take into account the changes in their operating environments. This self-adaptation is necessary to preserve the classifier performance. In this paper, the problem of designing classifiers in changing environments will be studied and the methods to design this type of classifiers will be presented and analyzed.
Read this article from a comprehensive knowledge base, updated and supplemented with articles reviewed by scientific committees.
Read the articleAUTHOR
-
Moamar SAYED MOUCHAWEH: Professor, Institut Mines-Telecom, École des Mines de Douai, Computer Science and Control Department
INTRODUCTION
Pattern Recognition (PR) covers all algorithms and methods for classifying patterns into classes. A shape is a simplified observation of a system's state, represented by a set of parameters. These parameters form the representation space that characterizes the system's operation or behavior. Each class is associated with a state or mode characterizing the system's behavior or operating conditions. The classification of a shape is performed by a classification module, called a classifier, using a rule or decision function. The problem of RoF is therefore to characterize the model and label of each class associated with a shape. This requires the use of classification techniques that enable similar shapes to be grouped together by learning.
Classes can be static or dynamic. Static classes are based on stationary data. This means that the parameters and model structure of each class remain unchanged over time. However, as the environment is constantly changing, most real-world data is non-stationary. Non-stationarity is defined as an evolution over time of the parameters and structure of the model or classifier due, for example, to wear and tear or aging of the machine, or to temporal changes in the characteristics of the system's environment. This non-stationarity modifies the static or dynamic behavior of the system, affecting the performance (predictive accuracy) of its model or classifier. Classes in this case are dynamic. The parameters and structure of the model or classifier must be updated to maintain its performance over time.
In this paper, the problem of learning models or classifiers in a non-stationary environment is studied. Suitable methods and techniques for learning effective classifiers for this type of environment are presented and analyzed. Predicting the occurrence of faults, filtering information (documents, e-mails), classifying documents and modeling user profiles and monitoring their evolution over time are just some of the areas of application of these methods.
Exclusive to subscribers. 97% yet to be discovered!
You do not have access to this resource.
Click here to request your free trial access!
Already subscribed? Log in!
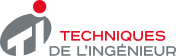
The Ultimate Scientific and Technical Reference
KEYWORDS
automatic learning | dynamic classification | drift detection | non-stationary environment | Information technology | engineerings | artificial intelligence | pattern recognition
This article is included in
Software technologies and System architectures
This offer includes:
Knowledge Base
Updated and enriched with articles validated by our scientific committees
Services
A set of exclusive tools to complement the resources
Practical Path
Operational and didactic, to guarantee the acquisition of transversal skills
Doc & Quiz
Interactive articles with quizzes, for constructive reading
Dynamic learning in a non-stationary environment
Bibliography
Exclusive to subscribers. 97% yet to be discovered!
You do not have access to this resource.
Click here to request your free trial access!
Already subscribed? Log in!
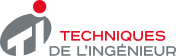
The Ultimate Scientific and Technical Reference