Overview

ABSTRACT
This synthesis presents the recent evolution of techniques for the evaluation and improvement of data quality in databases based on machine learning methods. It describes recent solutions proposed mainly by the academia as well as approaches implemented to detect and correct main data quality problems such as outlying, inconsistent or missing data, and duplicates.
Read this article from a comprehensive knowledge base, updated and supplemented with articles reviewed by scientific committees.
Read the articleAUTHOR
-
Laure BERTI-ÉQUILLE: Research Director - Development Research Institute - ESPACE-DEV - Montpellier, France
INTRODUCTION
Significant progress has been made in recent years in the design of tools to automate the evaluation, monitoring and improvement of data quality, thanks in particular to technological advances in Artificial Intelligence, and in particular machine learning (ML – Machine Learning). Machine learning techniques have been made operational on a large scale, and are now widely deployed in all sectors of activity, to automate prediction and classification tasks in decision support for numerous fields of application (health, finance, marketing, etc.). However, the reliability of these methods' results remains highly dependent on the quality of the input data for the learning models. Data is often imperfect, and optimal data quality is rarely achieved. Thus, two complementary approaches are commonly proposed: one from the data management research community, aimed at correcting data upstream of analysis chains (by cleaning or repairing data), and the other from the community of learning researchers and practitioners (data scientists), aimed at developing models that are more robust to noise and more efficient, with greater emphasis on transforming and preparing data for a particular predictive task.
For decades, for the data management community, data cleansing has consisted in correcting and transforming data using declarative ETL (Extraction-Transformation-Loading) approaches , detecting inconsistencies in relational databases in the form of constraint violations, to "repair" them and to propose solutions, often theoretical, enabling reasoning from inconsistent data, querying it, verifying and satisfying integrity constraints , discovering functional dependencies or business rules...
Exclusive to subscribers. 97% yet to be discovered!
You do not have access to this resource.
Click here to request your free trial access!
Already subscribed? Log in!
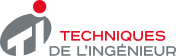
The Ultimate Scientific and Technical Reference
KEYWORDS
machine learning | data quality | data science | anomaly detection | data cleaning | data quality management | data repair
This article is included in
Software technologies and System architectures
This offer includes:
Knowledge Base
Updated and enriched with articles validated by our scientific committees
Services
A set of exclusive tools to complement the resources
Practical Path
Operational and didactic, to guarantee the acquisition of transversal skills
Doc & Quiz
Interactive articles with quizzes, for constructive reading
Detecting and correcting data quality problems using machine learning
Bibliography
Events
International conferences :
Very Large Databases (VLDB) Conference: http://vldb.org/conference.html
ACM SIGMOD (Special Interest Group on Management of Data): https://dl.acm.org/event.cfm?id=RE227
...
Standards and norms
- Data quality — Part 1: Overview https://www.iso.org/standard/50798.html - ISO/TS 8000-1 - 2011
- Data quality — Part 2: Vocabulary https://www.iso.org/standard/73456.html - ISO 8000-2 - 2017
- Data quality — Part 8: Information and data quality: Concepts and measuring https://www.iso.org/standard/60805.html - ISO 8000-8 - 2015
- Data quality — Part 61: Data quality management: Process reference model...
Exclusive to subscribers. 97% yet to be discovered!
You do not have access to this resource.
Click here to request your free trial access!
Already subscribed? Log in!
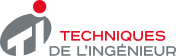
The Ultimate Scientific and Technical Reference