Overview

ABSTRACT
Medical images are often affected by random noise occurring in the image acquisition, measurement and transmission process. The resolution of this problem can lead to improved diagnostic and surgical procedures. Wavelet filtering can allow for a reasonably efficient noise reduction from the viewpoint of the average square error, SNR (signal-to-noise ratio) or PSNR (peak signal-to-noise ratio) and also in terms of visual quality.
Read this article from a comprehensive knowledge base, updated and supplemented with articles reviewed by scientific committees.
Read the articleAUTHOR
-
Abdeldjalil OUAHABI: Doctor of Engineering Grenoble INP, Doctor of Science - University Professor at Polytech'Tours (University of Tours)
INTRODUCTION
Medical imaging has revolutionized medical practice, becoming a commonly used tool in medicine. However, medical images are often affected by noise of a random nature, the source of which lies in the acquisition process. Solving this problem can lead to improved diagnoses and surgical procedures. It is therefore essential to eliminate this noise in order to enhance and recover the finest details that may be totally masked in noisy data.
The image filtering approach we propose here consists in converting the noisy image, initially observed in the spatial domain, into an image represented in the transformed domain, such as the wavelet or contourlet domain. The resulting wavelet coefficients are then compared with a fixed or adaptive threshold.
The current boom in wavelet transforms is mainly due to two specific properties resulting from decompositions on orthogonal wavelet bases: representation sparsity and the tendency to transform a stationary random process into decorrelated Gaussian sequences.
In the context of noise reduction, more commonly known as "denoising", the success of wavelet-based multiresolution analysis is precisely ensured by its decorrelation capability (separation of noise and useful signal) and by the notion of parsimony in its representation.
This parsimony is materialized by a small number of high-amplitude wavelet coefficients (or, more precisely, wavelet transform coefficients) representing the useful signal, assumed to be regular or piecewise regular. Noise, often assumed to be white and stationary, will tend to be spread over all wavelet components or coefficients.
Relying on these two properties (sparsity and decorrelation), appropriate filtering in the wavelet domain and calculation of the corresponding inverse wavelet transform will yield the denoised signal.
In general, image denoising using wavelet-based multi-resolution analysis requires a compromise between noise reduction and the preservation of significant image detail.
The performance of this filtering will be analyzed, both in terms of mean square error and signal-to-noise ratio (SNR or PSNR), and in terms of visual quality in the case of images.
This article is a follow-up to
Exclusive to subscribers. 97% yet to be discovered!
You do not have access to this resource.
Click here to request your free trial access!
Already subscribed? Log in!
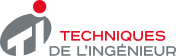
The Ultimate Scientific and Technical Reference
KEYWORDS
denoising | wavelets | multiresolution | implementation | wavelets | electronics | biomedical | telecommunications | medical imaging | systems | measurements | random signal analysis | image processing
CAN BE ALSO FOUND IN:
Home IT Signal processing and its applications Wavelet digital filtering - Applications in medical imaging
Home Biomedical - pharma Healthcare technologies Wavelet digital filtering - Applications in medical imaging
Home Measurements - Analysis Electronic measurements and tests Wavelet digital filtering - Applications in medical imaging
This article is included in
Electronics
This offer includes:
Knowledge Base
Updated and enriched with articles validated by our scientific committees
Services
A set of exclusive tools to complement the resources
Practical Path
Operational and didactic, to guarantee the acquisition of transversal skills
Doc & Quiz
Interactive articles with quizzes, for constructive reading
Wavelet-based digital filtering
Bibliography
Exclusive to subscribers. 97% yet to be discovered!
You do not have access to this resource.
Click here to request your free trial access!
Already subscribed? Log in!
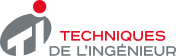
The Ultimate Scientific and Technical Reference