Overview

Read this article from a comprehensive knowledge base, updated and supplemented with articles reviewed by scientific committees.
Read the articleAUTHOR
-
Gilles ZWINGELSTEIN: Engineer from the École nationale supérieure d'électrotechnique, d'électronique, d'informatique et d'hydraulique et des télécommunications de Toulouse (ENSEEIHT), Doctor of Engineering, Doctor of Science, retired Associate Professor of Universities, Université Paris-Est
INTRODUCTION
For operators of industrial processes, monitoring the state of health of their equipment is one of the major preoccupations in avoiding plant downtime. With this in mind, numerous methods and tools have been developed over the last few decades to detect the onset of deterioration, perform diagnostics and estimate the remaining service life before failure (DEFAD, RUL). The vast majority of them rely exclusively on experience and knowledge, data or physical models. This article presents a state-of-the-art review of hybrid methods that combine the advantages of the previous methods for failure diagnosis and prognosis. To avoid any ambiguity in the terms used in this article, the first section presents the main definitions and terminologies proposed by ISO and NF-EN international standards (ISO 13372:2012, ISO 13379-1 ISO 13381-1, ISO 16079-1 and NF EN 13306). The second section, after a brief reminder of the issues involved and the main steps involved, presents the general principles of hybrid methods. This is followed by a brief review of the main features of the elementary experience- and knowledge-based methods, data-based methods and physical model-based methods required to develop hybrid methods. As the various hybrid methods described in the literature are based on the serial or parallel combination of the preceding elementary methods, the third section presents their classification into five categories proposed by Linxia Liao. This typology takes into account: 1) methods based on experience, knowledge and data; 2) methods based on experience, knowledge and physics-based models; 3) methods based on multiple data processing; 4) methods based on data and physics-based models; 5) methods based on experience, knowledge, data and physics-based models.
To illustrate the diversity of hybrid approaches and their effectiveness, the fourth section presents four case studies. The first case concerns a hybrid method using data and physical models to predict the erosion of a choke valve in a subsea oil facility. It implements a procedure that uses data smoothing by autoregressive models, a standard neural network and a reliability model based on the Gamma law. The second case proposes a method for predicting the reliability of a ball bearing, using several data processing methods (principal component analysis, PCA and logistic regression). As the reliability of lithium-ion batteries plays an important role in the storage and supply of electrical energy, the third case proposes a method for predicting the charge capacity of a lithium battery, based on ARMA autoregressive models and neural networks. The last case proposes a method for modeling crack propagation speed without knowledge of propagation laws, with a view to RUL prediction. It uses a hybrid method based on a representation model and a neural...
Exclusive to subscribers. 97% yet to be discovered!
You do not have access to this resource.
Click here to request your free trial access!
Already subscribed? Log in!
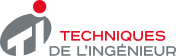
The Ultimate Scientific and Technical Reference
This article is included in
Maintenance
This offer includes:
Knowledge Base
Updated and enriched with articles validated by our scientific committees
Services
A set of exclusive tools to complement the resources
Practical Path
Operational and didactic, to guarantee the acquisition of transversal skills
Doc & Quiz
Interactive articles with quizzes, for constructive reading
Hybrid diagnostic and prognostic methods
Bibliography
Websites
The Prognostics and Health Management Society (PHM Society)
University of Cincinatti bearing test database site: NSF I/UCRC on Intelligent Maintenance Systems (IMS)
https://www.iucrc.org/center/nsf-iucrc-intelligent-maintenance-systems
...Standards and norms
- Machine condition monitoring and diagnostics – Vocabulary - ISO 13372 - 2012
- Condition monitoring and diagnostics of machines – data interpretation and diagnostics techniques – Part 1: General guidelines - ISO13379-1 - (2012)
- Machine monitoring and diagnostics – Prognosis – Part 1: General guidelines - ISO 13381-1 - (2015)
- Condition monitoring and diagnostics of wind turbines – Part 1: General...
Exclusive to subscribers. 97% yet to be discovered!
You do not have access to this resource.
Click here to request your free trial access!
Already subscribed? Log in!
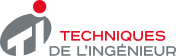
The Ultimate Scientific and Technical Reference