Overview

ABSTRACT
This article lists the inverse problems, be they well- or ill-posed, as well as the necessary tools for their diagnosis (conditioning and covariance, inversion test, influence of the number of measurements, etc.). By identifying six possible causes of estimation errors, the means to obtain a satisfactory inversion are explained. After having presented and compared certain popular regularization methods, this article offers a specific method of model reduction by identification. To conclude, the choice of the structure of a reduced model, its decomposition, identification, reconstitution and usefulness are dealt with via a linear case.
Read this article from a comprehensive knowledge base, updated and supplemented with articles reviewed by scientific committees.
Read the articleAUTHORS
-
Daniel PETIT: Professor at the École nationale supérieure de mécanique et d'aérotechnique ENSMA - Laboratoire d'Études Thermiques LET, UMR CNRS 6608
-
Denis MAILLET: Professor at the Institut national polytechnique de Lorraine INPL - Laboratory of energetics and theoretical and applied mechanics LEMTA, Nancy University & CNRS
INTRODUCTION
In the previous
In this dossier, we first return to the notions of a well-posed or poorly posed inverse problem, by outlining approaches and tools designed to better characterize this aspect of inversion. This involves quantifying the degree of seriousness of this characteristic ("poorly posed"), by adopting one or more criteria. We then highlight six possible causes of estimation error. These causes go beyond the mathematical aspects of the problem under consideration. Tools for diagnosing inversion quality are then proposed.
The notion of regularizing an ill-posed inverse problem, with the necessary trade-off between estimator dispersion and bias, is then introduced. Some popular regularization methods are then presented. They are applied and compared on the same example.
We also introduce the Bayesian approach, allowing additional external information of varying accuracy to be added to measurement inversion. Finally, a particular method of model reduction by identification is presented. It is based on input and output data obtained either from measurements or from simulations based on a detailed reference model. The first case corresponds to experimental model building, a procedure that can be likened to system calibration. In the second case, a model reduction technique is used, based on the principle of parsimony, to synthetically link only useful inputs and outputs. In both cases, the reduced model constructed can then be used to estimate inputs from outputs.
Exclusive to subscribers. 97% yet to be discovered!
You do not have access to this resource.
Click here to request your free trial access!
Already subscribed? Log in!
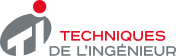
The Ultimate Scientific and Technical Reference
This article is included in
Physics and chemistry
This offer includes:
Knowledge Base
Updated and enriched with articles validated by our scientific committees
Services
A set of exclusive tools to complement the resources
Practical Path
Operational and didactic, to guarantee the acquisition of transversal skills
Doc & Quiz
Interactive articles with quizzes, for constructive reading
Inverse techniques and parameter estimation. Part 2
Bibliography
Exclusive to subscribers. 97% yet to be discovered!
You do not have access to this resource.
Click here to request your free trial access!
Already subscribed? Log in!
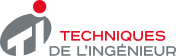
The Ultimate Scientific and Technical Reference