
5. Conclusion and outlook
This article has presented the fundamental principles of physics-inspired statistical learning. This is a very broad field, characterized by the use of physics concepts in conjunction with data-driven learning methods to address physical problems. These so-called "hybrid" methods are an emerging topic of major interest to many scientific communities. Physics can be incorporated into model learning in several ways: through appropriate selection of training data, in the form of soft constraints in the loss function, as hard constraints in neural network architectures, or in a modular fashion. From a learning perspective, these physical constraints enable the development of more interpretable models that conform to physical laws and remain robust in the presence of noisy data. This typically translates into greater efficiency in data utilization and better extrapolation performance beyond...
Exclusive to subscribers. 97% yet to be discovered!
You do not have access to this resource.
Click here to request your free trial access!
Already subscribed? Log in!
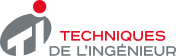
The Ultimate Scientific and Technical Reference
This article is included in
Technological innovations
This offer includes:
Knowledge Base
Updated and enriched with articles validated by our scientific committees
Services
A set of exclusive tools to complement the resources
Practical Path
Operational and didactic, to guarantee the acquisition of transversal skills
Doc & Quiz
Interactive articles with quizzes, for constructive reading
Conclusion and outlook
Bibliography
Exclusive to subscribers. 97% yet to be discovered!
You do not have access to this resource.
Click here to request your free trial access!
Already subscribed? Log in!
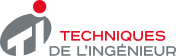
The Ultimate Scientific and Technical Reference