
2. Principal component analysis (PCA)
2.1 Objectives
The purpose of principal component analysis is to study data resulting from the observation of p quantitative variables on n individuals, arranged in a matrix X (n x p). The objectives are :
the "optimal" graphical representation of the individuals (lines), minimizing the distortions of the point cloud, in a subspace E q of dimension q (q < p) of the vector space
Exclusive to subscribers. 97% yet to be discovered!
You do not have access to this resource.
Click here to request your free trial access!
Already subscribed? Log in!
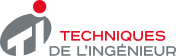
The Ultimate Scientific and Technical Reference
This article is included in
Software technologies and System architectures
This offer includes:
Knowledge Base
Updated and enriched with articles validated by our scientific committees
Services
A set of exclusive tools to complement the resources
Practical Path
Operational and didactic, to guarantee the acquisition of transversal skills
Doc & Quiz
Interactive articles with quizzes, for constructive reading
Principal component analysis (PCA)
Bibliography
Websites
Other resources (handouts, practical exercises, functions written in R) are available on the website :
https://www.math.univ-toulouse.fr/
R Development Core TeamR: A Language and Environment for Statistical Computing, R Foundation for Statistical Computing
Find out more
The most useful general and introductory references for this theme are: Bouroche & Saporta (1980), Jobson (1992), Lebart, Morineau & Piron (2006), Mardia, Kent & Bibby (1979), Saporta (2006). More recent additions and developments can be found in: Droesbeke, Fichet & Tassi (1992), Govaert (2003).
Exclusive to subscribers. 97% yet to be discovered!
You do not have access to this resource.
Click here to request your free trial access!
Already subscribed? Log in!
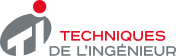
The Ultimate Scientific and Technical Reference